Sonic Kayaks are rigged with sensors, both underwater (temperature, sound, and turbidity) and above water (air pollution). As the kayaker paddles around, the sensors pick up changes in the environment, and these are played to the kayaker in real time through an on-board speaker. Originally this approach came from a sound art project (Kaffe Matthews’ Sonic Bikes), but we realised it could also be useful for researchers to be able to follow data or seek out particular environments through sound, and along the way we gathered interest from the visually impaired community for using the sounds to augment their kayaking experience or guide navigation on the water.
The Sonic Kayak project has been running for about 5 years now, but we’ve never had a chance to spend any real time developing the environmental data sonification approach. When Covid-19 came about, we had to adapt our ACTION funded project to replace participatory kayaking workshops, so we decided instead to ask for people’s help with the sonifications via an online survey. This approach takes us out of the trap of simply using the sonifications we prefer, means we can take a step back and see what actually works best for the most people, and gives more people a way to feed into the project as a whole. That said, this approach will probably annoy everyone equally but differently - we are trying to quantify something that was originally an artistic endeavour (which will be uncomfortable for some), but not analysing it scientifically as it is just for our own guidance (which will aggravate others).
The bulk of the effort came from sound artist Kaffe Matthews, who developed four approaches to sonifying the data, which we refer to as (i) synthesised sounds, (ii) robotic voice sounds, (iii) animal sounds, and (iv) granular animal sounds. For each approach, there needed to be three separate sonifications, one for the temperature sensor data, one for the turbidity data, and one for the air pollution data. The sounds have to work well together (sound palatable, but also distinct enough that people can tell between them), and mesh well with the live underwater sounds from the hydrophone and the ambient sounds from kayaking. This is no easy task.
Once we’d agreed on the four sonification approaches, we made a mock data set, and a video for each sonification to expose people to how they worked. For each video, you first hear each sensor’s sonification in turn (1 min), then all three sensor’s sonifications together (1 min). This meant we could ask people (1) could they tell between the three sounds, and (2) did they like listening to them together. The videos have background sounds of paddling, the water, birds, and people talking, and the visuals are of a kayak on the water – these were to provide a more realistic context for the sonifications. You can watch those videos here:
We also wanted to test whether people could tell what was happening with the data just based on the sonifications. For this we made a test video for each of the four sonification approaches, where each sensor was doing something different – either the data was rising, falling, rising then falling, or falling then rising – and asked people to pick which they though was happening for each sensor. All three sensors were being sonified at the same time, so it made for a very difficult task - we hoped this would give us some indication as to which were easiest to interpret. You can watch those videos here:
This was all set up as an online survey, and we had it checked over by blind audio and accessibility expert Gavin Griffiths (Power Audio Productions) to make sure it would work for people with visual impairments who use screen readers. To help us navigate the results, we asked one basic demographic question at the start of the survey – whether the respondant previously/currently works in scientific research, previously/currently works in a sound related job, is a musician (professional or hobby), and/or has a visual impairment.
The results from the 49 participants are available in full and are summarised below. We considered everyone’s results together, but also took a look at these subgroups:
- Those who selected ‘I work or have worked in scientific research’ but not ‘I work or have worked in a sound-related job’ or ‘I am a musician (either professional or hobby)’ (n = 14)
- Those who selected either ‘I work or have worked in a sound-related job’ or ‘I am a musician (either professional or hobby)’ but not ‘I work or have worked in scientific research’ (n = 9)
- Those who selected both ‘I work or have worked in scientific research’ and either ‘I work or have worked in a sound-related job’ or ‘I am a musician (either professional or hobby)’ (n = 9).
- Those who selected ‘I have a visual impairment’ (n = 2).
Given the small numbers in each of these subgroups, we need to treat this just as guidance not as necessarily meaningful results.
Can you confidently make out the three different sonifications?
Considering all participants together, people were the most confident with the Synthesised sonifications (yes = 34, I think so = 15, I don’t think so = 0, no = 0) and the Robotic Voice sonifications (yes = 45, I think so = 2, I don’t think so = 0, no = 2). People were less confident with the Animal (yes = 22, I think so = 16, I don’t think so = 10, no = 1) and Granular Animal (yes = 14, I think so = 13, I don’t think so = 15, no = 7) sonifications. When we broke the results down by demographic, this result was basically consistent across the board.
Did you find the sounds enjoyable to listen to?
Participants were asked to rank the sounds from 1 (not at all enjoyable) to 5 (very enjoyable). The enjoyment of sound is very subjective, and we expected a lot of split opinions here. Generally speaking, the sounds that people preferred were the Animal sonifications (mean score = 3.53) followed by the Synthesised sonifications (mean score = 3.41). The Robotic Voice sonifications scored the worst (mean score = 2.24), which we expected because they are very repetitive and dry to listen to, and the Granular Animal sonifications also scored low (mean score = 2.51). Again, when we broke the results down by demographic, this result was basically consistent across the board.
Could people tell what was happening with the data just by listening?
This was the real test – regardless of how confidently people thought they could tell between the sounds, or how enjoyable they were to listen to, the sonifications need to give people a clear idea of what is happening in the data.
The sounds that overall people got the most right for were the Synthesised sonifications (73% correct answers), and the Robotic Voice sonifications (73% correct answers). The proportion of correct answers was much lower for the Animal sonifications (39%) and very low for the Granular Animal sonifications (7%).
Splitting the results up by demographic once again showed that this result is broadly consistent across the different groups – but there were some interesting hints in the data:
- The people who selected ‘sound-related job’ or ‘musician’ were much better at the Synthesised sound tests (81% correct) than the Robotic Voice sound tests (70% correct), while all other groups scored equally for these.
- The people who selected both ‘sound-related job’ or ‘musician’ AND ‘scientific research job’ were incredibly good at the Synthesised sound tests (89% correct)
- When looking at the 4 demographic groups separately, two groups did better with the Synthesised sonifications than the Robotic Voice sonifications, and the other two groups were equally good at the Synthesised and Robotic Voice sonifications – so if we want to pick a sonification approach that works well for the most types of people, probably the Synthesised sounds are best.
Some quotes
Just to demonstrate some of the strength of disagreements for the same sounds, and some of the trade-offs (and also because they made us laugh):
Synthesised sounds: “A little uncomfortable. A bit like horror movie sound effects. The paddling in the water and birds did help to make it calmer, though.” vs. “Sounded intuitive. Continuous fluid (temp); and particles both clear (airborne) and muffled (submarine).”
Robotic Voice sounds: “Stressful to listen to. I wouldn't be able to listen to this for more than a minute before finding it really irritating.” vs. “ it was clearer to disentangle the voices and understand what was happening. I think you could soon learn to understand the data”
Animal sounds: “I heard seagulls in the first clip which was confusing. I think the sounds are too close to natural sounds to contrast with the environment.” and “Annoying fluttering I keep slapping my ear and looking round” vs “This one was sweet to listen to and seemed more intuitive.” and “Loved it! :)”
Granular Animal sounds: “Musically I'm drawn to sounds like these anyway and that's influencing my choice and my translation of the sounds The sounds are quite abstract and I'm drawn to start to think if more familiar sounds were used that the participant wouldn't be directed they would rather be guided into their own translation” vs. “Am genuinely surprised at how much my dislike of the chitinous insect swarm sound distracted me from what was going on. Shivers, like nails on a blackboard.”
What happens next
We’ll use these results to make decisions about which sonifications to use. In the short term, we’ll most likely use the Synthesised sounds, because they seem to offer the best compromise between enjoyability and interpretability. In the long term we might try to work more on the animal sounds, which people generally found most enjoyable, to make them easier to interpret. We also need to try the sounds out in real life, on the water - the setting can change everything...
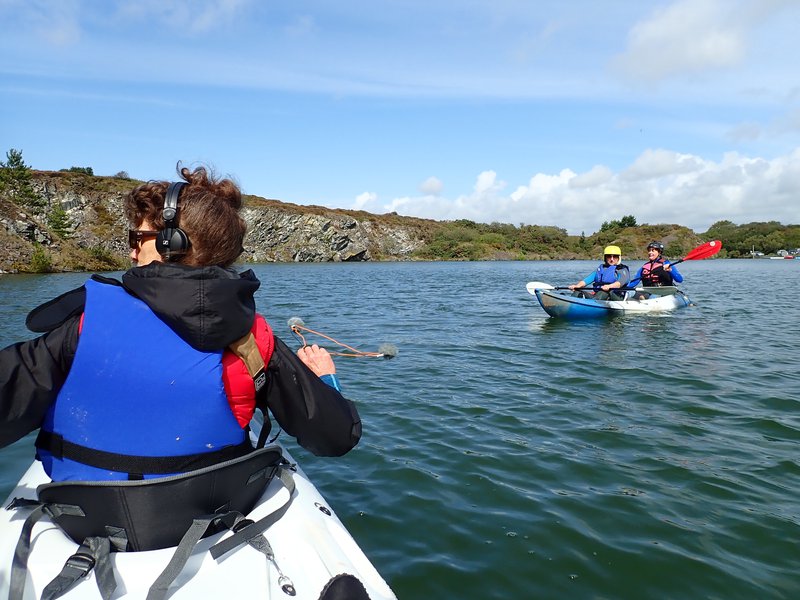